Speaker Details
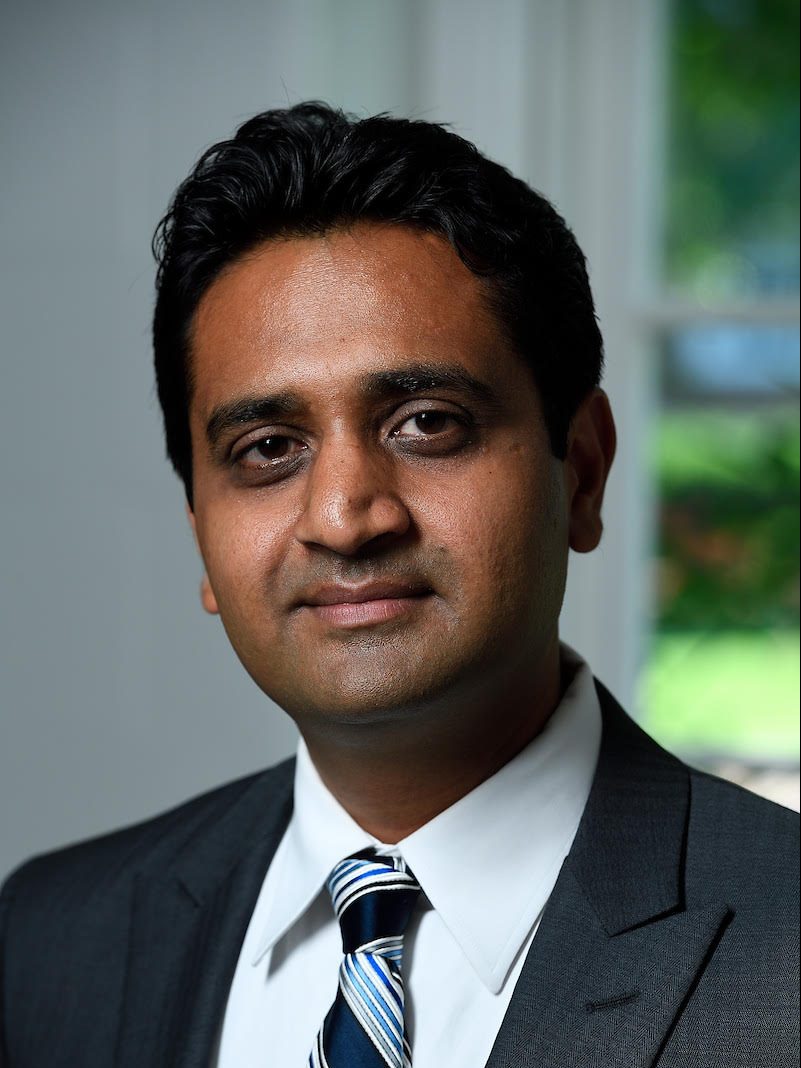
Vishal M. Patel
John Hopkins University
Bio:
Vishal M. Patel is an Assistant Professor in the Department of Electrical and Computer Engineering (ECE) at Johns Hopkins University. Prior to joining Hopkins, he was an A. Walter Tyson Assistant Professor in the Department of ECE at Rutgers University and a member of the research faculty at the University of Maryland Institute for Advanced Computer Studies (UMIACS). His current research interests include signal processing, computer vision, and pattern recognition with applications in biometrics and imaging. He has received a number of awards including the 2016 ONR Young Investigator Award, the 2016 Jimmy Lin Award for Invention, A. Walter Tyson Assistant Professorship Award, Best Paper Award at IEEE AVSS 2017, Best Paper Award at IEEE AVSS 2019, Best Paper Award at IEEE BTAS 2015, Honorable Mention Paper Award at IAPR ICB 2018, two Best Student Paper Awards at IAPR ICPR 2018, and Best Poster Awards at BTAS 2015 and 2016. He is an Associate Editor of the IEEE Signal Processing Magazine, IEEE Biometrics Compendium, Pattern Recognition Journal, and serves on the Information Forensics and Security Technical Committee of the IEEE Signal Processing Society. He is serving as the Vice President (Conferences) of the IEEE Biometrics Council.
Keynote Title:
Synthetic to Real Transfer Learning for Single Image Deraining
Keynote Abstract:
Recent CNN-based methods for image deraining have achieved excellent performance in terms of reconstruction error as well as visual quality. However, these methods are limited in the sense that they can be trained only on fully labeled data. Due to various challenges in obtaining real world fully-labeled image deraining datasets, existing methods are trained only on synthetically generated data and hence, generalize poorly to real-world images. The use of real-world data in training image deraining networks is relatively less explored in the literature. In this talk, I will present a Gaussian Process-based semi-supervised learning framework which enables the network in learning to derain using synthetic dataset while generalizing better using unlabeled real-world images.
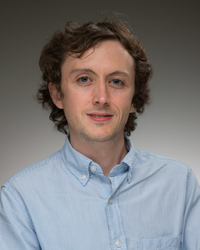
Walter J. Scheirer
University of Notre Dame
Bio:
Walter J. Scheirer, Ph.D. is an Assistant Professor in the Department of Computer Science and Engineering at the University of Notre Dame. Previously, he was a postdoctoral fellow at Harvard University, with affiliations in the School of Engineering and Applied Sciences, Dept. of Molecular and Cellular Biology and Center for Brain Science, and the director of research & development at Securics, Inc., an early stage company producing innovative biometrics solutions. He received his Ph.D. from the University of Colorado and his M.S. and B.A. degrees from Lehigh University.
Dr. Scheirer has extensive experience in the areas of human biometrics, computer vision, machine learning and artificial intelligence. His overarching research interest is the fundamental problem of recognition, including the representations and algorithms supporting solutions to it. He has made important contributions to the field of biometrics through his work on open set recognition, extreme value theory statistics for visual recognition, and template protection. His recent work has explored the intersection between neuroscience and computer science, leading to new, biologically-informed, ways to evaluate and improve algorithms.
He is very active within the biometrics and computer vision communities, having served as the program chair of IEEE/IAPR IJCB, IEEE WACV, and the SPIE Conference on Biometric and Surveillance Technology for Human and Activity Identification. Dr. Scheirer is also a regular organizer of IEEE/CVF CVPR, and sits on the board of the Computer Vision Foundation.
Keynote Title:
Visual Psychophysics for Making Face Recognition Algorithms More Explainable
Keynote Abstract:
Scientific fields that are interested in faces have developed their own sets of concepts and procedures for understanding how a target model system (be it a person or algorithm) perceives a face under varying conditions. In computer vision, this has largely been in the form of dataset evaluation for recognition tasks where summary statistics are used to measure progress. While aggregate performance has continued to improve, understanding individual causes of failure has been difficult, as it is not always clear why a particular face fails to be recognized, or why an impostor is recognized by an algorithm. Importantly, other fields studying vision have addressed this via the use of visual psychophysics: the controlled manipulation of stimuli and careful study of the responses they evoke in a model system. In this talk, we suggest that visual psychophysics is a viable methodology for making face recognition algorithms more explainable, including the ability to tease out bias. A comprehensive set of procedures is developed for assessing face recognition algorithm behavior, which is then deployed over state-of-the-art convolutional neural networks and more basic, yet still widely used, shallow and handcrafted feature-based approaches.
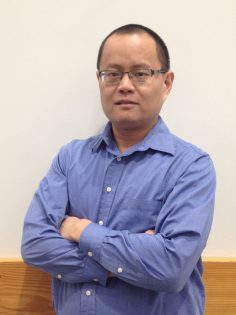
Yang Wang
University of Manitoba
Bio:
Yang Wang is an Assistant Professor in the Department of Computer Science at the University of Manitoba. He graduated with a Ph.D. from Simon Fraser University, where he was advised by Prof. Greg Mori. He received his M.Sc. from University of Alberta, and his B.Sc. from Harbin Institute of Technology. Before joining the Department of Computer Science at the University of Manitoba in July 2012, He worked as an NSERC postdoc fellow at UIUC with Prof. David Forsyth. His group works on a variety of topics in computer vision, machine learning, deep learning. He received the 2017 Falconer Emerging Researcher Rh Award in applied science and hold the inaugural Faculty of Science research chair in fundamental science (2019-2022).
Keynote Title:
Learning Video Summarization with Limited Data
Keynote Abstract:
With the large amount of videos available online, video summarization has become an important topic in computer vision. Given the input long video, the goal of video summarization is to produce a shorter video that contains the main content of the original video. In this talk, I will present several of our recent work on using deep learning for video summarization, especially in cases where we do not have enough labeled data, e.g. learning from unpaired videos, learning personalized model, etc.
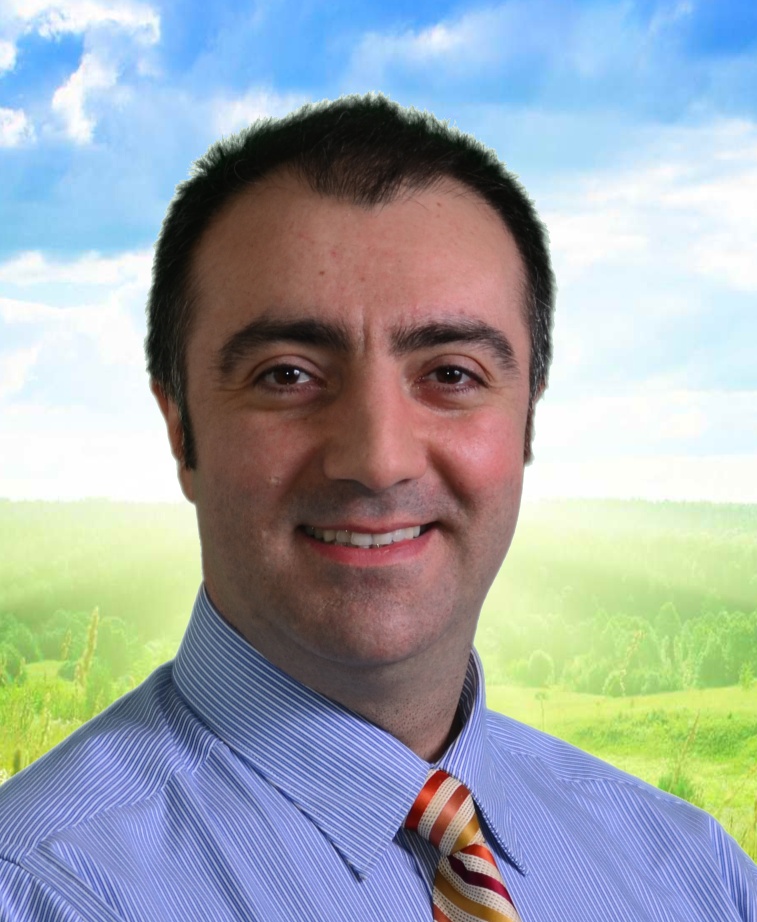
Fatih Porikli
Australian National University
Bio:
Fatih Porikli is an IEEE Fellow and a Professor in the Research School of Engineering, Australian National University (ANU). He has received his Ph.D. degree from New York University (NYU) in 2002. He served as the Vice President of the Futurewei (Huawei USA) Device & Hardware in San Diego. He led the Computer Vision Research Group Leader at NICTA, Australia and managed projects as the Distinguished Research Scientist at Mitsubishi Electric Research Laboratories, Cambridge. He developed satellite imaging solutions at HRL, Malibu CA, and 3D display systems at AT&T Research Laboratories, Middletown, NJ. His research interests include computer vision, deep learning, manifold learning, online learning, and image enhancement with commercial applications in mobile phones, AR/VR, autonomous vehicles, video surveillance, defense, and medical systems. He received the R&D 100 Scientist of the Year Award in 2006, won six best paper awards, and recognized with six professional prizes at his industrial appointments. He authored more than 250 publications, co-edited two books, and invented 80 US patents. He organized several IEEE conferences as General Chair and Technical Program Chair for the past 15 years. He is an Associate Editor of premier IEEE and Springer journals.
Keynote Title:
When Data Dictates the Problem
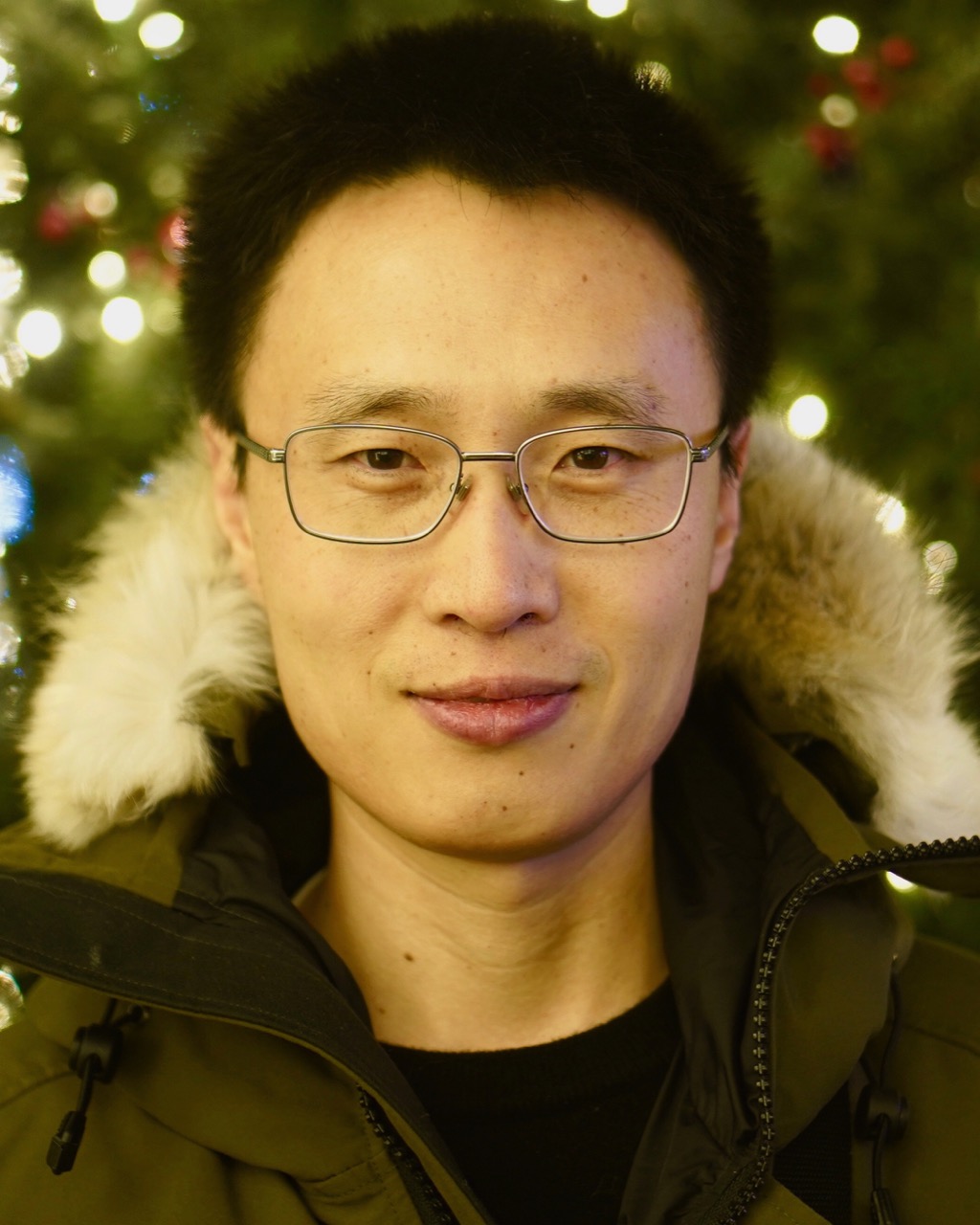
Boqing Gong
Bio:
Boqing Gong is a research scientist at Google, Seattle and a principal investigator at ICSI, Berkeley. His research in machine learning and computer vision focuses on sample-efficient learning (e.g., domain adaptation, few-shot, reinforcement, webly-supervised, and self-supervised learning) and the visual analytics of objects, scenes, human activities, and their attributes. Before joining Google in 2019, he worked in Tencent and was a tenure-track Assistant Professor at the University of Central Florida (UCF). He received an NSF CRII award in 2016 and an NSF BIGDATA award in 2017, both of which were the first of their kinds ever granted to UCF. He is/was a (senior) area chair of CVPR, ICCV, ECCV, NeurIPS, ICML, AISTATS, WACV, and AAAI. He received his Ph.D. in 2015 at the University of Southern California, where the Viterbi Fellowship partially supported his work.
Keynote Title:
Rethinking Class-Balanced Methods for Long-Tailed Visual Recognition from A Domain Adaptation Perspective
Keynote Abstract:
Object frequency in the real world often follows a power law, leading to a mismatch between long-tailed training sets seen by a machine learning model and our expectation of the model to perform well on all classes. We analyze this mismatch from a domain adaptation point of view. First of all, we connect existing class-balanced methods for long-tailed classification to target shift, a well studied assumption in domain adaptation. The connection reveals that these methods implicitly assume that the training and test data share the same class-conditioned distribution, which does not hold in general and especially for the tail classes. Indeed, while a head class could contain rich and diverse training examples that well represent the expected data at inference time, the tail classes are often short of representative training data. To this end, we propose to augment the classic class-balanced learning by explicitly estimating the differences between the class-conditioned distributions with a meta-learning approach. We validate our approach on six benchmark datasets and three losses.
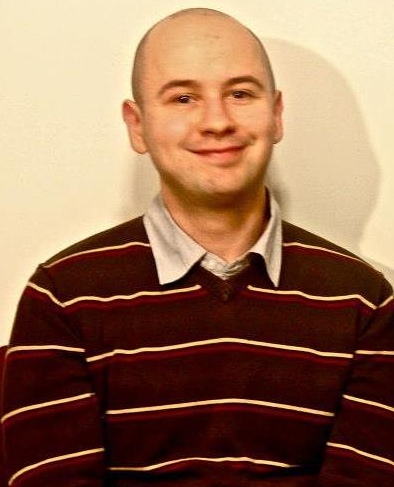
Piotr Koniusz
Australian National University
Bio:
Dr. Piotr Koniusz is a senior researcher in Machine Learning Research Group at Data61/CSIRO (former NICTA). He is also a senior honorary lecturer at Australian National University (ANU). Previously, he worked as a post-doctoral researcher in the team LEAR, INRIA, France. He received his BSc degree in Telecommunications and Software Engineering in 2004 from the Warsaw University of Technology, Poland, and completed his PhD degree in Computer Vision in 2013 at CVSSP, University of Surrey, UK.
His interests include visual concept detection, visual category recognition, action recognition, zero-, one- and few shot learning, domain adaptation, image-to-image translation, feature and representation learning, invariance learning and understanding, feature pooling, spectral learning and graphs, as well as tensor, kernel methods, linearisations, sparsity and deep learning methods.
Keynote Title:
Statistical Representations for Domain Adaptation and Few-shot Learning